In recent years, the finance industry has undergone profound transformations, with artificial intelligence (AI) and machine learning (ML) emerging as key drivers of change. These technologies are reshaping the way financial institutions operate, make critical decisions, and engage with their customers. From improving efficiency to enhancing decision-making processes, ML is making its mark across various aspects of finance.
In this blog post, we’ll dive into the growing role of machine learning within the finance sector. We’ll explore real-world applications, examine case studies, and highlight some of the specific ML models that are powering this shift.
However, it’s important to recognize that while machine learning holds immense potential, its impact is not universal. The effectiveness of ML can vary greatly depending on the context, application, and the way it is implemented. As we delve into this topic, we’ll provide a balanced perspective, weighing both the significant benefits and the challenges that come with integrating machine learning into financial services.
The Adoption of Machine Learning in Finance
Machine learning, a branch of artificial intelligence, is rapidly gaining traction in the finance industry due to its remarkable ability to analyze vast amounts of data, identify intricate patterns, and make data-driven predictions. Several key factors are driving this growing adoption:
- • Increased Data Availability: The rise of digital technologies has led to an explosion of financial data, offering a rich source of information for machine learning algorithms to process and learn from.
- • Advancements in Computing Power: The continued evolution of computing technology, including powerful hardware and cloud infrastructure, has made it more practical and cost-effective to run complex ML models at scale.
- • Regulatory Changes: In the wake of the 2008 financial crisis, the need for more robust and sophisticated risk management tools has intensified. ML has emerged as a promising solution to help financial institutions meet these new demands.
- • Competitive Pressures: Financial institutions are increasingly turning to ML to gain a competitive edge, leveraging it in areas such as algorithmic trading, personalized customer service, and product innovation.
In the following sections, we’ll explore specific applications of machine learning in finance, drawing on real-world case studies to highlight how the technology is being put to work. While these examples illustrate the promising potential of ML, it’s important to remember that the technology is still evolving, and its long-term impact on the industry is yet to be fully realized.
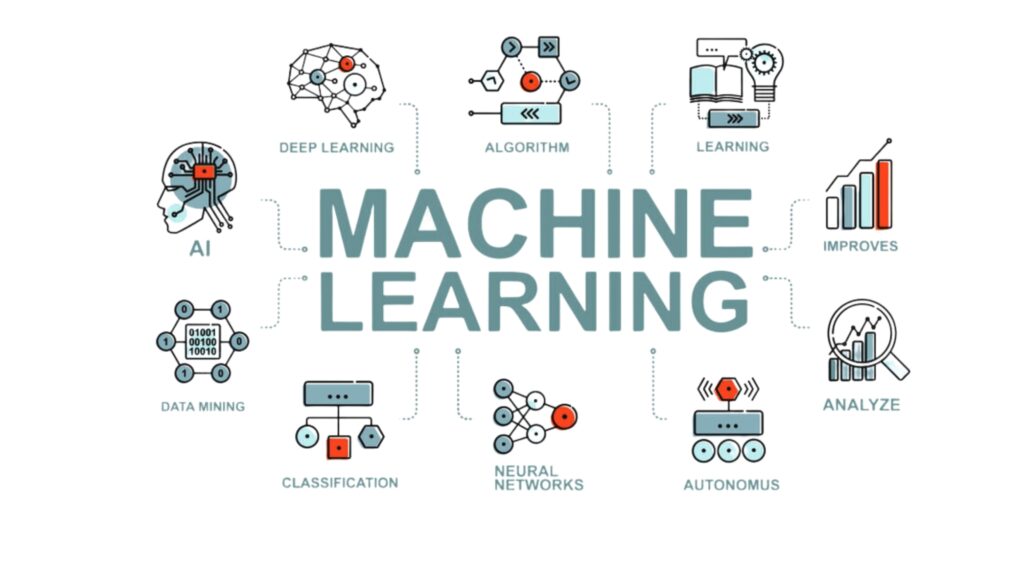
Document Analysis and Processing
- Reduced Document Review Time: COiN streamlined the process, cutting down review time from hundreds of thousands of hours to just seconds.
- Enhanced Accuracy: The platform significantly improved the accuracy of interpreting loan agreements, minimizing errors in the process.
- Lowered Operational Risk: By automating document review, COiN reduced the risk of human error and the associated operational risks.
- Natural Language Processing (NLP): NLP models enable the platform to understand and extract key insights from unstructured text data within legal documents.
- Named Entity Recognition (NER) and Part-of-Speech Tagging: These techniques help the platform identify and extract specific entities, such as borrower names, dates, and loan amounts, to make sense of complex legal language.
- Machine Learning Algorithms: The algorithms are trained on large sets of annotated examples, allowing COiN to continuously improve its understanding of legal terminology and document structure over time.
Risk Management and Portfolio Optimization
- Advanced Risk Assessment: Aladdin delivers highly accurate risk metrics across diverse investment portfolios, enabling more precise risk management.
- Actionable Insights for Portfolio Managers: By processing complex financial data, Aladdin empowers portfolio managers with deep, data-driven insights that inform strategic decisions.
- Global Asset Management at Scale: The platform manages trillions of dollars in assets across multiple markets and asset classes, supporting a wide range of investment strategies.
- Regression Analysis: Used to forecast asset performance and assess associated risk factors with precision.
- Clustering Algorithms: Groups assets and market conditions to identify patterns and correlations, enhancing portfolio diversification and strategy.
- Time-Series Forecasting: Provides predictive analytics on future market trends and asset performance, helping to anticipate market movements.
- Monte Carlo Simulations: Models portfolio behavior under various hypothetical market scenarios, allowing for robust scenario analysis and stress testing.
Fraud Detection and Security
- Enabled instantaneous identification and blocking of fraudulent transactions
- Improved customer experience by minimizing unnecessary transaction declines (reduced false positives)
- Continuously updated models to adapt to new fraud patterns and techniques
- Deep Learning Neural Networks: To handle high-dimensional data and capture complex, non-linear relationships between variables
- Ensemble Methods (Random Forests and Gradient Boosting): To improve predictive accuracy by combining multiple models
- Anomaly Detection Algorithms: To identify unusual patterns or outliers in transaction behavior
Algorithmic Trading and Investment Management
- Exceptional Returns: The Medallion Fund averaged an astounding 66% annual return before fees from 1988 to 2018, far surpassing traditional market indices and other hedge funds.
- Outperformance: The fund consistently outperformed both broader market indices and peers in the hedge fund industry, demonstrating its unique edge in trading.
- Hidden Markov Models (HMM): These models are likely employed to identify latent states in financial markets, enabling the prediction of price movements based on unobservable market conditions.
- Neural Networks: Used for advanced pattern recognition and non-linear modeling, neural networks help capture complex relationships in market behavior that traditional models often miss.
- Reinforcement Learning: This technique likely powers the development of adaptive, self-improving trading strategies that evolve over time, optimizing decisions in real-time based on market feedback.
Customer Service and Personalization
- Rapid Adoption: Over 17 million users have engaged with Erica since its launch in 2018.
- High Volume of Interactions: In its first two years, Erica processed more than 100 million client requests, demonstrating its scalability and efficiency.
- Increased Engagement & Satisfaction: Erica has driven significant improvements in customer engagement, providing on-demand assistance and increasing overall satisfaction.
- Natural Language Processing (NLP): Enables Erica to understand and respond to customer queries in natural, conversational language, enhancing user interaction.
- Sentiment Analysis: Assesses the emotional tone of customer communications, allowing Erica to tailor responses based on the customer’s mood and urgency.
- Predictive Analytics: Leverages individual customer data to provide proactive, personalized financial advice, anticipating needs and recommending actions before they are explicitly asked for.
Credit Scoring and Financial Inclusion
- Increased Access to Credit: ZestFinance has enabled financial institutions to extend credit to underserved and previously excluded markets, broadening financial inclusion.
- Lower Default Rates: By improving risk assessment, ZestFinance has helped reduce default rates, enabling more accurate prediction of borrower risk.
- Regulatory Compliance: The company provides transparent and explainable credit models that adhere to lending regulations, ensuring trust and legal compliance.
- Gradient Boosting Machines (GBMs): Utilized for analyzing structured data and capturing complex, non-linear relationships between borrower attributes and creditworthiness.
- Ensemble Learning: By combining multiple weak models into a stronger predictive system, ZestFinance enhances the overall accuracy and robustness of its credit scoring models.
- Feature Engineering and Selection: The process of carefully selecting the most predictive features from a large pool of potential variables, optimizing the model’s ability to identify key factors that influence creditworthiness.
Challenges and Future Outlook
Machine learning has undoubtedly brought transformative benefits to the finance industry, but it also introduces a range of challenges that need to be carefully addressed:
- Data Privacy and Security Concerns: The massive amounts of personal and financial data used in machine learning models raise significant privacy and security risks.
- Regulatory Compliance: Ensuring that AI/ML applications meet evolving regulatory standards is an ongoing challenge for financial institutions.
- The “Black Box” Problem: Many advanced machine learning models operate as opaque systems, making it difficult to understand how decisions are made, which can undermine trust.
- Algorithmic Biases: Unintended biases in data or models can lead to unfair outcomes, particularly in credit scoring, lending, and hiring decisions.
Despite these challenges, the future of machine learning in finance remains highly promising, with several key trends on the horizon:
- Sophisticated AI-Driven Financial Products: We can expect increasingly personalized and intelligent financial products that offer tailored solutions for individuals and businesses.
- Advancements in Explainable AI: To address the “black box” problem, we will likely see greater adoption of explainable AI models, making decision-making processes more transparent and understandable.
- Integration of Alternative Data: Financial institutions will increasingly rely on non-traditional data sources (e.g., social media activity, transaction data, and geolocation) to enhance the accuracy and robustness of machine learning models.
- Enhanced Natural Language Processing: Advances in NLP will improve customer service by enabling more intuitive and responsive AI-driven interactions, from chatbots to voice assistants.
As these case studies demonstrate, machine learning is already making a significant impact across multiple areas of finance, from enhancing risk management and fraud detection to enabling personalized customer service and driving financial inclusion.
Looking forward, we can expect more innovative applications that will continue to reshape the industry. Financial institutions that successfully adopt and integrate machine learning technologies are likely to gain a competitive edge in an increasingly tech-driven environment. The convergence of finance and AI marks a pivotal shift in how financial services are delivered, and it will undoubtedly play a key role in shaping the future of the sector.